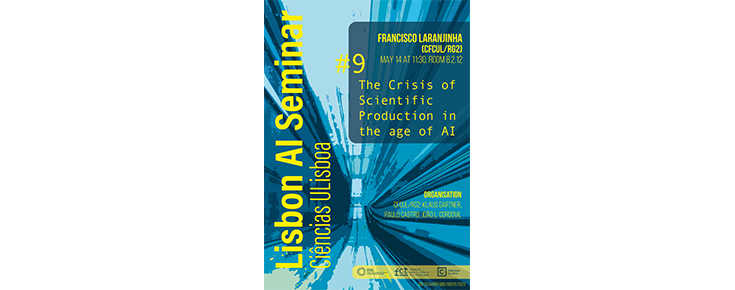
Por Francisco Laranjinha (CFCUL/RG2).
Many applications of scientific theories often involve the use of computational models because, in many case studies, the equations that describe a given physical system do not have an analytical solution. For physical systems whose differential equations either do not have analytical solutions or the analytical solution is extremely complicated, usually a numerical solution implemented in a simulation provides some form of insight into the physical system in study. However, when we consider Machine learning we are considering a whole different form of computational model. A standard definition of Machine learning would be a subset of AI that ‘learns’ to make decisions by fitting mathematical models to observed data. A deep neural network is a type of machine learning model that is referred to as deep learning when it is fitted to data. It is a very different form of computational model given its autonomy and learning capabilities. In this talk, I will try to explain some of the problems this brings when we talk about scientific discovery. If computers can learn or think (whatever that is) then we must ask the question; will they be able to produce new scientific discoveries autonomously? I propose to look at this question as a derivation of Turing Imitation Game. The Scientific Imitation Game. Furthermore, I argue that, if we look at scientific practice, we can form a very strong intuition that AI driven scientific discoveries will be the next scientific revolution.